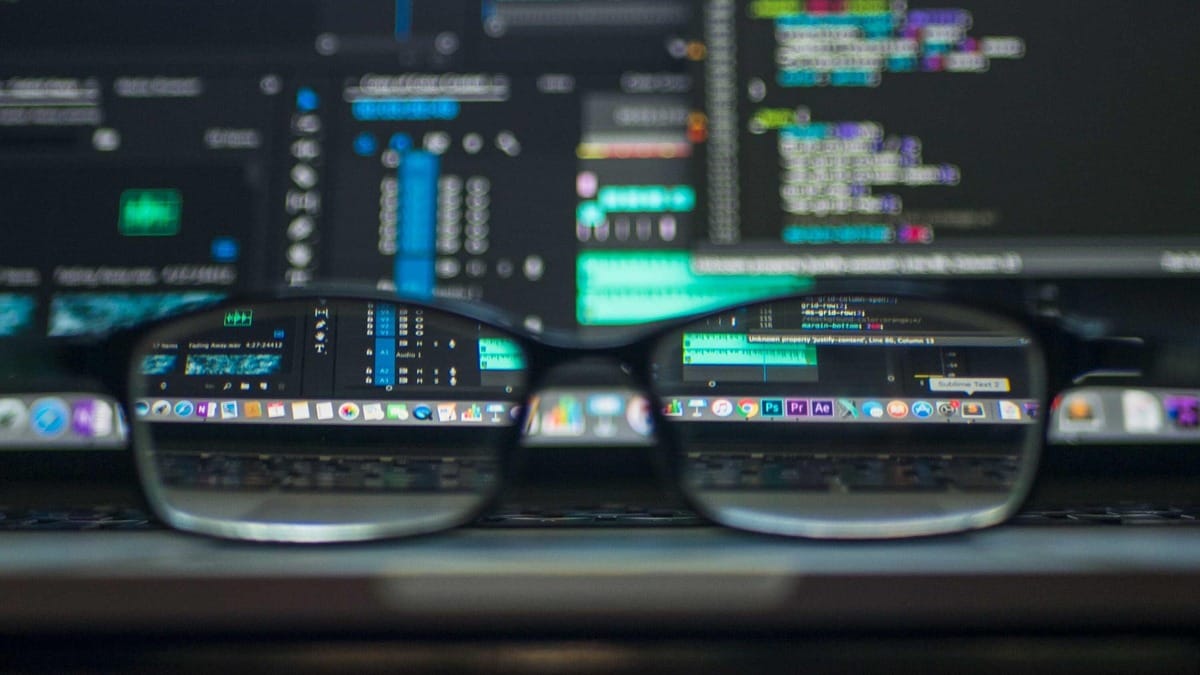
Reclaiming human agency in how we think about AI
Reading Time: 9 minutes Online panic about AI models like ChatGPT follows a well-travelled path set by impoverished understandings of evolutionary theory. Can we reclaim human agency?
Like many, I’ve been disheartened by recent human response to augmented or artificial intelligence, as it becomes a hot topic in everything from art and freelance writing, to programming, to legal counsel, to scientific research. I’ve written thrice already on this theme: how our future shock around this tech is age-old economic anxiety, how corporate monopolies are the real problem, and why we need to detangle human value from careerist potential. Nevertheless, there is an abiding thread of helplessness, of passive reaction to various reported feats of AI, that runs so deep in mainstream media and social media reaction as to frustrate this humanist immensely.
So let’s talk about the limiting nature of determinist thinking. Yes, AI is here, but the way we tell stories about it cordons off possible futures, and denies us better, more ethical, and even more empowering uses of the technology. We’re so caught up in how it might ruin specific careers (as ever so many new processes and technologies, along with corporate downsizing and cost-cutting measures, have done before) that we’re accepting replacement theory as the dominant mode for discourse around these new mechanical toolsets.
It doesn’t have to be that way.
But let’s take a look, first, at what in our culture makes us fall prey to such simplistic beliefs at all.
Determinist thinking in the secular world
When it comes to the great debate between evolutionary psychology and evolutionary biology, I come down firmly on the side of the biologists. Evolutionary psychology surged at the turn of the 20th century, and continues to inform how many analyze human behaviour: namely, by reading back from existing interactions to how they might have been selected during evolutionary processes for “enhanced inclusive fitness” (Robert Foley, 1995). But the problem with this whole approach is twofold: one, it fails to offer effective checks against attitudinal biases on the part of the researcher, often producing “just so” stories based on contemporary mores read back through deep time; and two, it makes a complete hash of evolutionary processes.
As Elizabeth Anne Lloyd and Marcus W Feldman explained during the peak of evo-psych fervor:
One result of the presentation of evolutionary theory as equivalent to inclusive fitness theory is that it focuses all attention on adaptation as a result of optimization of inclusive fitness, which in many cases is not the best way to represent the evolutionary dynamic (Cavalli-Sforza & Feldman, 1978). This is especially true, for example, in cases of sexual or fertility selection, where fitness is properly assigned to a mating pair rather than individual genotypes. When these forces are stronger than the forces of differential survival ability, predictions made by maximizing inclusive fitness will not yield correct results. Indeed, under these modes of selection, the mean fitness of the population is not maximized by the process of evolution at all.
“Evolutionary Psychology: A View from Evolutionary Biology”, Psychological Inquiry, April 2002
Put simply, evolutionary psychology often trips into teleology, treating evolution as an efficient driver of species into streamlined forms, and handwaves over matters of scale around key sites of evolutionary action. If folks act a certain way today, even if it’s maladaptive in its current context, it must have been adaptive at some point, right? There must have been a purpose, a peak performance, that this trait once represented?
Except that’s not the way evolutionary processes work, which is why the field of evolutionary biology, which wades deep into the messy evidence given to us by evolutionary history, has always been the more robust body of data for me (a non-scientist, and interested layperson) when trying to make sense of the world. Give me Robert Sapolsky explaining why maladaptive and adaption-neutral traits are a normative side-effect of gene selection that just often enough also produces adaptive behaviours, in lieu of of saying that male shooters, say, are all an extension of an adaptive behaviour in the past, which just isn’t fitting as well into the modern age.
(Down that road lies the nonsense buoying the Jordan Petersons of the world, too.)
In this, I’m also fortified by my own academic background in histories of literature, with an emphasis on histories of literary science: namely, how we’ve told stories about ourselves, and of our discoveries in the natural world, over time. These critical touchstones have allowed me to move with much more comfort through the idea that “science” is not monolith, and that scientists are not objective selectors of their fields of research, nor of their approach to the research done.
This in turn means that the core principles of scientific method (falsification and replication) only ever resolve for individual error over time. If we let them. If the myth of singular genius, or the compelling nature of a given scientific story, hasn’t made presenting a proper challenge to it a Herculean task. (See: the complex institutional mess made of mathematical physics, as described by Lee Smolin and Sabine Hossenfelder.) I’ve also seen, through literary studies in general, how our cultural mores have changed over the centuries, so I’m much quicker than some to spot when a scientific story today is comfortably upholding contemporary myths.
(STEAM, not STEM, folks: history matters!)
One pointed example comes from Angus J. Bateman’s famed 1948 fruit fly study, which was used for decades to justify the idea that men are naturally more promiscuous and women are naturally more “choosy”. Now, any student of medieval history should be laughing at this claim wholesale, because in other eras it was women who were considered the more sexually unreliable, the more easily overcome by their carnal urges, and the more likely to lead good, upright men astray when they weren’t off cavorting with the Devil himself, if not strictly controlled through the firm guiding hand of (men of) faith.
Sadly, we still have much of that rhetoric today, in stigma around single mothers as people who must have wantonly pursued casual sex with no regard for possible outcomes, and through constant penalizing of feminine students’ attire in schools, as wilfully leading boys and men on. But somehow those counterpoints haven’t cut short the nonsense idea that men are naturally more promiscuous.
We are large; our species contains multitudes of cognitive dissonance.
The scientific gap
But surprisingly, considering its widespread importance to the field, Bateman’s fly study went fairly uncontested, and unreplicated, for decades. It was just such an obvious finding! Of course women, “natural” homemakers and mothers, would far prefer a single partner, if multiple partners didn’t grant them the ability to produce more offspring. And of course men, “natural” adventurers and conquerors, would far prefer multiple partners, if it granted them the ability to seed widely. And even if the study itself was only ever dealing with fruit flies, surely the results would graft seamlessly onto humans!
Then in 2012, Patricia Adair Gowaty (et al.) published the results of the first attempt to replicate Bateman’s findings using his methodology. “No evidence of sexual selection in a repetition of Bateman’s classic study of Drosophila melanogaster“ (Proceedings of the National Academy of Sciences) didn’t just fail to reproduce Bateman’s findings; it also highlighted the glaring flaws in initial methodology that should have raised red flags a lot sooner. The most important involved the fact that Bateman was doing his research before DNA testing would allow for easy identification of parentage; absent this tool, he’d selected fly populations with distinct traits, like narrow eyes and curled wings. Then he only counted the offspring with two distinct traits: the ones from which he could identify maternal and paternal lineage.
Not only did this mean that all the offspring with only one (or no) visible traits were excluded, it also meant that his sample set was reduced by the fact that two extreme mutations reduces viability. He was working with extremely limited data, even for flies. And when the full populations were counted, with tools in a modern lab? The supposed difference in mating strategy success rates could not be replicated.
There are many other such misguided examples in recent history. There’s also the Stanford prison experiment, which everyone loves to use as an “obvious” confirmation of Hobbesian ideas that man’s basic nature is nasty, but which involved researchers expressly coaxing participants to be more brutal than was their natural inclination, among other procedural failings. Then there’s Rosenhan’s 1973 paper, which undermined psychiatric assessment by “proving” that a person could fake an illness and get treated as seriously as if they really had it (except that Rosenhan’s pseudo-patients couldn’t be sourced, and the ability to trick a doctor doesn’t preclude the existence of real cases on which they’re basing their interpretation of symptoms).
And this isn’t even taking into account a broader recent critique of psychological studies, as only representing WEIRD (Western, Educated, Industrial, Rich, and Democratic) countries, and of frequently drawing from small, select sample sets (e.g. university students) that for many reasons shouldn’t be considered adequate reflections of the whole species. Major rehauls in how we talk about and “do” science are necessary, to curtail the existence of such errors.
The connection to AI
All of which is to say that received wisdom is often difficult for us to question. When an argument is presented that accords with existing beliefs and cultural norms, we lean into it more readily than arguments that contravene “common knowledge”. Plus, we just love a good factoid that affirms our current choices, don’t we? What’s that? A study says that dark chocolate and red wine are good for us? Sign us up!
And so it goes with how we’re responding to AI news now.
AI keeps getting treated in mainstream and social media like a body of literal competitors with human beings: alt-humans, like ever so many robots and non-human sentiences we’ve been given through sci-fi for decades.
ChatGPT, the algorithmic model developed to “converse” in a way that produces fairly coherent content, is especially being treated as a kind of impending HAL 9000 or Skynet, because this is the cultural backdrop against which we’re trying to make sense of new, related tech.
And yet, all this program does is feed back to us its best guesses for the correct follow-up to the content we’ve provided, based on a synthesis of all the human-made inputs it’s been given to date. If it’s accurate at all, it’s because the baseline data humans first disseminated was accurate. And when it’s in error? Sometimes that’s because it still cannot work out answers even to basic logic problems. Other times, it’s because the most common human responses are in error, too.
Our impoverished evolutionary theory is also showing, in the determinism we give over to when talking about such algorithms as our inevitable inheritors. After all, they’re streamlined forms of us, aren’t they? Just look at how much more efficiently they can do so many of our tasks!
But that’s the wrong way to think about evolutionary processes. So let’s lean on the messiness given to us by sociobiology instead, and use that to reframe how we think about this latest tool: in a way, that is, which upholds human agency by admitting human limits.
Earlier this century, there was also a massive drive to talk about the problems of “Big Data”. Computer technology had raced so far ahead of human discourse that we were now dealing with an overwhelming amount of raw data, and very little sense of how to sift through and synthesize it all effectively. Of the many TED Talks alone that grappled with this theme, Shyam Sankar’s 2012 address made a salient point that we seem to have forgotten while discussing recent augmented intelligences: namely, that we’re never going to find one perfect algorithm to do all our work for us, but we can build better symbiotic relationships between humans and the tech we’ve created.
When I see ChatGPT in operation, I see it doing the work of synthesizing all text-based human knowledge (in English, from its provided data-sets) in a way that shows our current priorities. It’s reading our “pulse”, in other words: reflecting back to us the most frequent connections we humans currently make when talking about given themes. That’s why folks at OnlySky received pretty comprehensive descriptions of secular topics or questions of morality: because those conversations exist widely enough in the surrounding world as to be best-guess answers for the algorithm.
For certain topics, this allows such programs to provide us with a more comprehensive overview of a given issue than we might have come up with on our own. If you ask ChatGPT what a person should consider when choosing a university, for instance, its amalgam of all existing human-made prep material stands a good chance of covering more factors than any average respondent would.
Such tools also allow us to monitor the persistence of myths and general falsehoods, if we continue to remember our agency in this new symbiotic landscape. Because while ChatGPT and similar algorithms synthesize and regurgitate chunks of human-generated data on a given topic, the only way for us to recognize when this data is in error is to remain sharp in our own logical processing, and our own synthesis of the same materials. It’s only through careful human curation, after all, that ChatGPT hasn’t fallen victim to always spitting out violent, sexist, antisemitic, racist, and otherwise inappropriate remarks: a fate common for chat programs in the past, as they built new response sets from past interactions with vulgar and hateful users.
Yes, AI is here, but the way we tell stories about it cordons off possible futures, and denies us better, more ethical, and even more empowering uses of the technology.
The big ‘so what?’
In most every field of discovery, we humans have a tendency to accept, wholesale, whatever already fits neatly into received wisdom and cultural norms. When we throw in our impoverished understanding of evolutionary processes, winnowed down to a simplistic understanding of adaptive benefit and competitive fitness, it’s no wonder that we’ve given way in the last few months to wildly demoralizing talk about AI taking over the world.
But reframe this issue to include the full messiness of human society. Include the surfeit of “Big Data” we’ve been struggling to synthesize effectively for years. Allow for the corporate eagerness to cut costs and maximize profit that’s driving current AI hype and panic. Remember the many ways that human frivolity, vulgarity, and malevolent action have junked up existing data sets already. And don’t forget our inability to recognize subjectivity even when engaging in scientific processes.
Taken together, the cultural context surrounding the emergence of these next-gen tech tools is predicated on a great deal of human fragility and fallibility, which bleeds into all the systems and algorithms we create and uplift as well.
This is our evolutionary heritage.
Not streamlined efficiencies. Not teleological endpoints.
Just a mess of ever-shifting impressions and possibilities: some adaptive, but most less so. Most carried along, one generation after another, by the current of “good enough” first-draftism.
And in this context lies our strength—our agency—if we’ve half a mind to remember it.
The current received wisdom about AI, as given to us in so much of mainstream and social media, is not much different from ChatGPT’s own output: a regurgitation of knee-jerk best-guesses for the most reasonable response to “AI is here, and it’s coming for your field”.
But we can go beyond those knee-jerk best-guesses. We can sift through all the recent data on this tech and its contexts, and decide if that best-guess is the right one.
And we can choose to reframe our thinking—if and when we realize that it’s not.