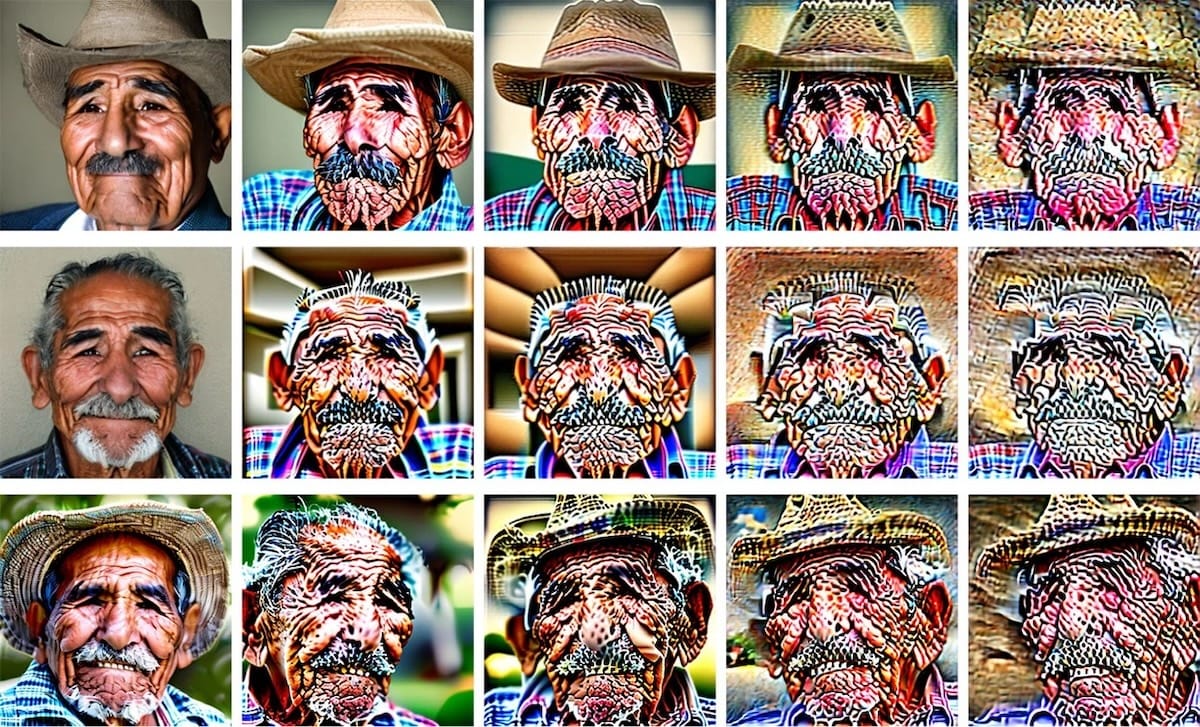
Model collapse—the end of the road for AI
AI is developing the same problem as cousins who marry—and for the same reason.
AI is running wild, drowning out human creators in a flood of synthetic text and images. This blizzard of slop may mean the death of the internet, at least the attention-economy-based, advertising-funded internet we know.
But AI technology is itself facing an existential threat, and ironically, it's a threat that's arisen because AI has been so successful. This looming iceberg goes by the name of model collapse.
AI chatbots and artbots are neural networks: software designed to mimic the web of connections between neurons in our brains. They "learn" by analyzing vast quantities of data and discovering statistical correlations. Chatbots learn that some words follow other words with either high or low probability, and they use that knowledge to generate sentences. Artbots learn that descriptions like "dog" or "airplane" or "America" correlate to characteristics of an image that feature detectors can respond to, and they can reverse that process to make images matching a prompt.
This worked amazingly well. The first generation of AIs had the whole canon of human creativity to learn from: billions of web pages, books, articles, images and photographs. Their creators scraped it all from the internet and poured it through the software brains of their creations. The copyright implications are debatable, but the results aren't. By crunching this river of data, AI bots learned how to answer questions, how to write code, how to make art, how to analyze images, and countless other clever tricks.
Of course, these bots aren't flawless. For all their talents, they sometimes generate garbled text, or false factual claims, or weirdly melted and deformed images. Their creators dismissed these as inevitable early bugs in a technology that's still maturing and improving. They promised that with more training data, AI would keep getting better, until it could not only match human performance but surpass it.
But there's a problem: the internet is no longer pristine. It's been polluted by immense quantities of texts and images generated by these AIs. There's no reliable way to screen this out, which means that later generations of AIs will be trained on data created by earlier generations of AIs. Because of this, AIs are no longer learning how to be more human; they're learning how to be more like AI.
You can think of this as the AI version of inbreeding—and it's a problem for the same reason that inbreeding is harmful in nature.
When cousins marry
Every living thing carries genes for a few rare, recessive disorders. Normally, the genetic reshuffling of sex minimizes the chances that these bad mutations manifest in the next generation.
But when two closely related organisms breed, the chance of their offspring inheriting these genes increases. Instead of diluting the unwanted mutations out of the population, they become more common. The more generations inbreeding goes on, the more numerous and worse the health problems become in each new generation. This "inbreeding depression" has been observed across species, from pedigree dogs to European royalty.
The same problem exists for AI. Every AI model contains spurious and incorrect correlations in its neural map. Those erroneous connections—or, if you prefer, false beliefs—cause hallucinations and other chronic problems with output. With enough human-made data to learn from, these glitches can be smoothed away. However, when AIs are trained on data created by other AIs, the errors multiply.
For a microcosm of the problem, consider artbots' infamous inability to draw hands and fingers. This happens because AIs don't inherently "know" that hands are supposed to have five fingers, each with different lengths, that can bend and move in certain ways but not others. They have to infer this from their training data.
But the millions of pictures with hands are all from different angles, with different lighting and varying levels of detail. Sometimes fingers are obscured by other objects. Sometimes hands are intertwined, and the AI model has no a priori way to know which finger goes with which person's body. Some images are of cartoon characters or other depictions with different numbers of fingers. It's no wonder artbots have such a hard time getting this right.
This is a hard enough problem as it is. Now imagine new AI artbots trained on old AI artbot output, featuring those Lovecraftian hands that look like twisted clumps of fingers. With these grotesque mistakes poisoning the pool of training data, even the already-low possibility of getting hands right will dwindle to zero.
Diminishing returns
You can extrapolate this problem across every domain where AI has been tried. Unless it can be trained only on data that's guaranteed to be human-created, model collapse is inescapable. Tech companies are going to increasingly extreme lengths to source more pristine data, but it's a curve of diminishing returns.
Model collapse doesn't mean that AI will cease to exist. However, it may well mean that it stops getting better. As opposed to techno-utopians who claim it's only a matter of time until AI becomes superintelligent, it may be about to hit a hard stop.
It would be a grand irony if this technology, which seemed to have so much promise at the outset, ends up eating its own waste and poisoning itself in the bargain.